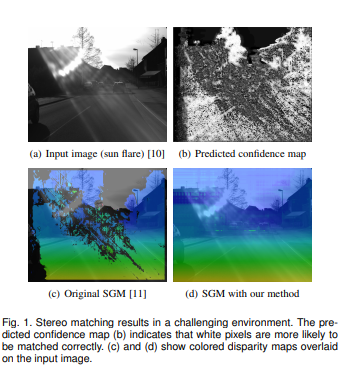
Learning and Selecting Confidence Measures for Robust Stereo Matching
– Published Date : TBD
– Category : Stereo Matching
– Place of publication : IEEE Transactions on Pattern Analysis and Machine Intelligence (TPAMI)
Abstract
We present a robust approach for computing disparity maps with a supervised learning-based confidence prediction. This approach takes into consideration following features. First, we analyze the characteristics of various confidence measures in the random forest framework to select effective confidence measures depending on the characteristics of the training data and matching strategies, such as similarity measures and parameters. We then train a random forest using the selected confidence measures to improve the efficiency of confidence prediction and to build a better prediction model. Second, we present a confidence-based matching cost modulation scheme, based on predicted confidence values, to improve the robustness and accuracy of the (semi-) global stereo matching algorithms. Finally, we apply the proposed modulation scheme to popularly used algorithms to make them robust against unexpected difficulties that could occur in an uncontrolled environment using challenging outdoor datasets. The proposed confidence measure selection and cost modulation schemes are experimentally verified from various perspectives using the KITTI and Middlebury datasets.