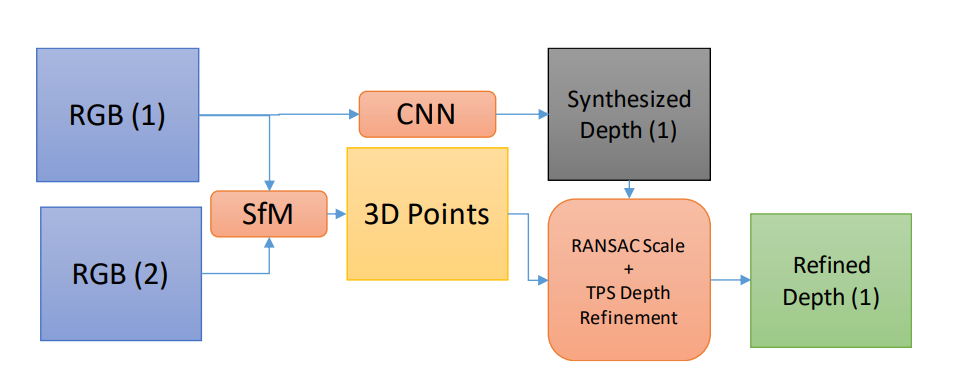
Refining Synthesized Depth from Deep Neural Networks for 3D Reconstruction
Abstract:
An intermediate field of interest which fuses the well-known area of 3D scene reconstruction and the reborn area of deep neural networks is synthesizing depth images from monocular or stereo cameras. One major weakness which is not thoroughly addressed is considering the ability of such algorithms in reconstructing the scene in a consistent manner, rather than only having nice shaped depths. To be more specific, a depth image synthesized from a monocular camera image using convolutional neural networks may show reasonable relative depth values for a single scene. But for a sequence of depth images from the same scene, the depth values are highly unstable temporally. This causes loss in the depth consistency and scale information, which results in inaccurate scene reconstruction. This paper addresses a way to refine raw synthesized depth outputs of convolutional neural fields consistently to be able to perform accurate 3D reconstruction. Our experiments support the idea by demonstrating well-structured scenes with high global accuracy comparable with the ground truth data.