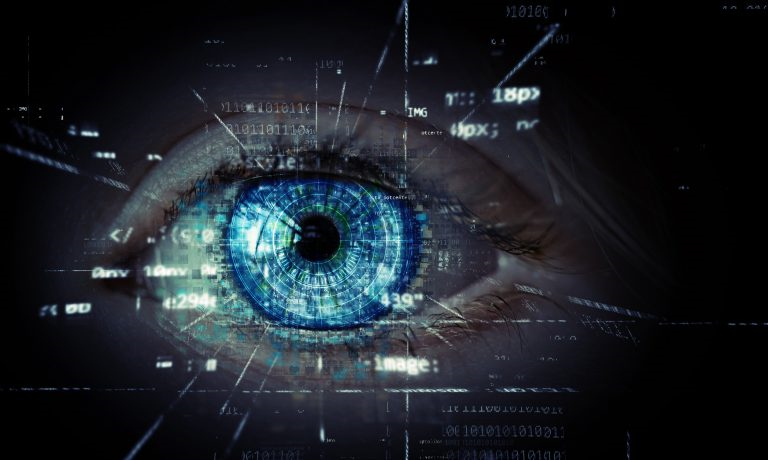
Gaussian Mixture Importance Sampling Function for Unscented SMC-PHD Filter
– Published Date : 2013
– Category : Tracking and Detection
– Place of publication : Signal Processing
Abstract
The unscented sequential Monte Carlo probability hypothesis density (USMC-PHD) filter has been proposed to improve the accuracy performance of the bootstrap SMC-PHD filter in cluttered environments. However, the USMC-PHD filter suffers from heavy computational complexity because the unscented information filter is assigned for every particle to approximate an importance sampling function. In this paper, we propose a Gaussian mixture form of the importance sampling function for the SMC-PHD filter to considerably reduce the computational complexity without performance degradation. Simulation results support that the proposed importance sampling function is effective in computational aspects compared with variants of SMC-PHD filters and competitive to the USMC-PHD filter in accuracy.