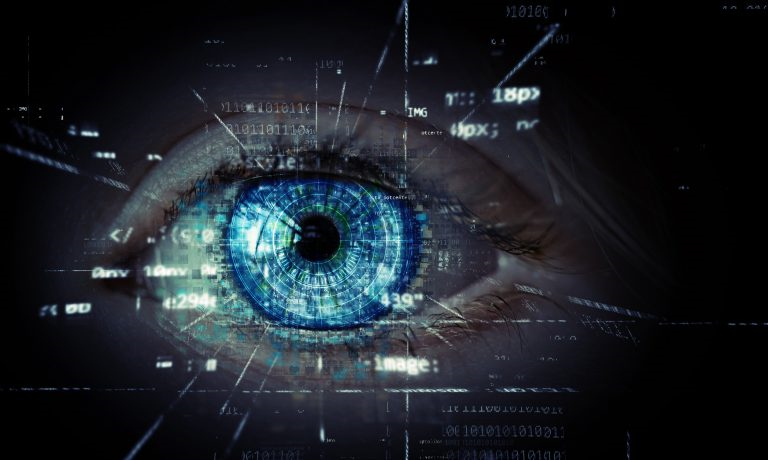
Multi-Object Tracking Using Hybrid Observation in PHD filter
– Published Date : 2013
– Category : Tracking and Detection
– Place of publication : International Conference on Image Processing (ICIP)
Abstract
In this paper, we propose a novel multi-object tracking method to track unknown number of objects with a single camera system. We design the tracking method via probability hypothesis density (PHD) filtering which considers multiple object states and their observations as random finite sets (RFSs). The PHD filter is capable of rejecting clutters, handling object appearances and disappearances, and estimating the trajectories of multiple objects in a unified framework. Although the PHD filter is robust to cluttered environment, it is vulnerable to missed detections. For this reason, we include local observations in an RFS of observation model. Local observations are locally generated near the individual tracks by using on-line trained local detector. The main purpose of the local observation is to handle the missed detections and to provide identity (label information) to each object in filtering procedure. The experimental results show that the proposed method robustly tracks multiple objects under practical situations.