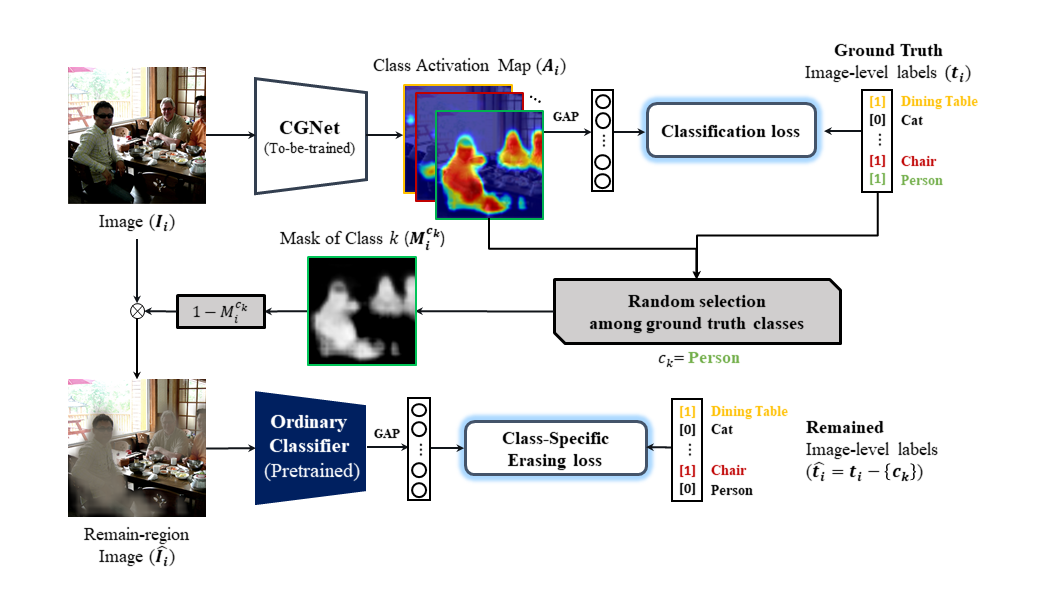
Unlocking the Potential of Ordinary Classifier: Class-specific Adversarial Erasing Framework for Weakly Supervised Semantic Segmentation
– Published Date : TBD
– Category : Weakly-supervised semantic segmentation
– Place of publication : IEEE/CVF International Conference on Computer Vision (ICCV) 2021
Abstract:
Weakly-supervised semantic segmentation (WSSS) usingimage-level classification labels usually utilizes the Class Activation Maps (CAMs) to localize objects of interest in images. While pointing out that CAMs only highlight the most discriminative regions of the classes of interest, adversarial erasing (AE) methods have been proposed to further explore the less discriminative regions. In this paper, we review the potential of the pre-trained classifier which is trained on the raw images. We experimentally verify that the ordinary classifier already has the capability to activatethe less discriminative regions if the most discriminative regions are erased to some extent. Based on that, we propose a class-specific AE-based framework that fully exploiting the potential of an ordinary classifier. Our framework (1) adopts the ordinary classifier to notify the regions to be erased and (2) generates a class-specific mask for erasing by randomly sampling a single specific class to be erased(target class) among the existing classes on the image for obtaining more precise CAMs. Specifically, with the guidance of the ordinary classifier, the proposed CAMs Generation Network (CGNet) is enforced to generate a CAM of the target class while constraining the CAM not to intrude the object regions of the other classes. Along with the pseudo-labels refined from our CAMs, we achieve the state-of-the-art WSSS performance on both PASCAL VOC 2012 and MS-COCO dataset only with image-level supervision.