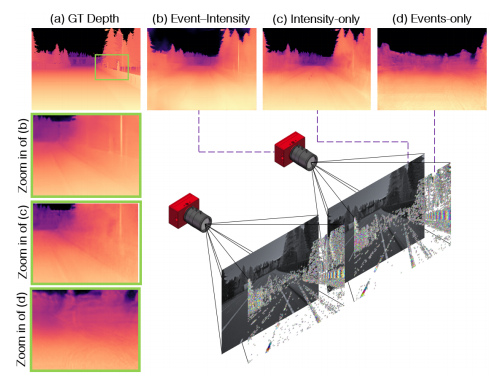
Event-Intensity Stereo: Estimating Depth by the Best of Both Worlds
– Published Date : TBD
– Category : Stereo matching
– Place of publication : IEEE/CVF International Conference on Computer Vision (ICCV) 2021
Abstract:
Event cameras can report scene movements as an asynchronous stream of data called the events. Unlike traditional cameras, event cameras have very low latency (microseconds vs milliseconds) very high dynamic range (140 dB vs 60 dB), and low power consumption as they report changes of a scene and not a complete frame. As they report per pixel feature-like events and not the whole intensity frame they are immune to motion blur. However, event cameras require movement between the scene and camera to fire events, i.e., they have no output when the scene is relatively static. Traditional cameras, however, report the whole frame of pixels at once in fixed intervals but have lower dynamic range and are prone to motion blur in case of rapid movements. We get the best from both worlds and use events and intensity images together in our complementary design and estimate dense disparity from this combination. The proposed end-to-end design combines events and images in a sequential manner and correlates them to estimate dense depth values. Our various experimental settings in real-world and simulated scenarios exploit the superiority of our method in predicting accurate depth values with fine details. We further extend our method to extreme cases as depth. The camera parameters and the stereo setup are mainly available through calibration, and the task is to triangulate the matched pairs to recover the disparity or depth [31]. What makes stereo matching challenging goes back to the ill-posed nature of the problem, occlusions, imperfect imaging settings, blurred or low dynamic range images, and also repetitive patterns or texture-less regions [21]. Recent methods estimate depth using learning-based frameworks that can also be trained end-to-end. Unlike previous methods, learning-based networks do not rely on hand-crafted parameters, and can also estimate metric depth based on the prior knowledge of the network. This is possible thanks to the modern GPUs, creative architectures, and public available large scale datasets. The main direction in many of the stereo depth estimation networks is reaching novel network architectures, further accuracy, faster predictions or fewer parameters. Al-of missing the left or right event or stereo pair and also investigate stereo depth estimation with inconsistent dynamic ranges on the left and right pairs.