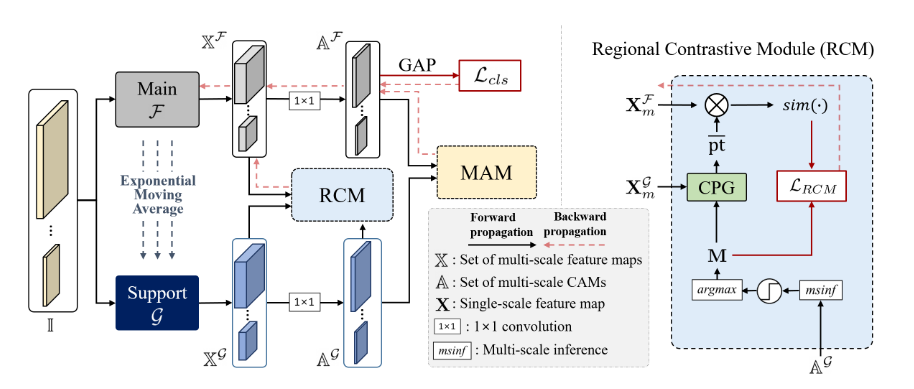
Exploring Pixel-level Self-supervision for Weakly Supervised Semantic Segmentation
– Published Date : 2022/02/09
– Category : Weakly Supervised Semantic Segmentation
– Place of publication : The 34th Workshop on Image Processing and Image Understanding (IPIU 2022)
Existing studies in weakly supervised semantic segmentation (WSSS) have utilized class activation maps (CAMs) to localize the class objects. However, since a classification loss is insufficient for providing precise object regions, CAMs tend to be biased towards discriminative patterns (i.e., sparseness) and do not provide precise object boundary information (i.e., impreciseness). To resolve these limitations, we propose a novel framework (composed of MainNet and SupportNet.) that derives pixel-level selfsupervision from given image-level supervision. In our framework, with the help of the proposed Regional Contrastive Module (RCM) and Multi-scale Attentive Module (MAM), MainNet is trained by self-supervision from the SupportNet. The RCM extracts two forms of selfsupervision from SupportNet: (1) class region masks generated from the CAMs and (2) class-wise prototypes obtained from the features according to the class region masks. Then, every pixel-wise feature ofthe MainNet is trained by the prototype in a contrastive manner, sharpening the resulting CAMs. The MAM utilizes CAMs inferred at multiple scales from the SupportNet as self-supervision to guide the MainNet. Based on the dissimilarity between the multiscale CAMs from MainNet and SupportNet, CAMs from the MainNet are trained to expand to the less-discriminative regions. The proposed method shows state-of-the-art WSSS performance both on the train and validation sets on the PASCAL VOC 2012 dataset. For reproducibility, code will be available publicly soon.