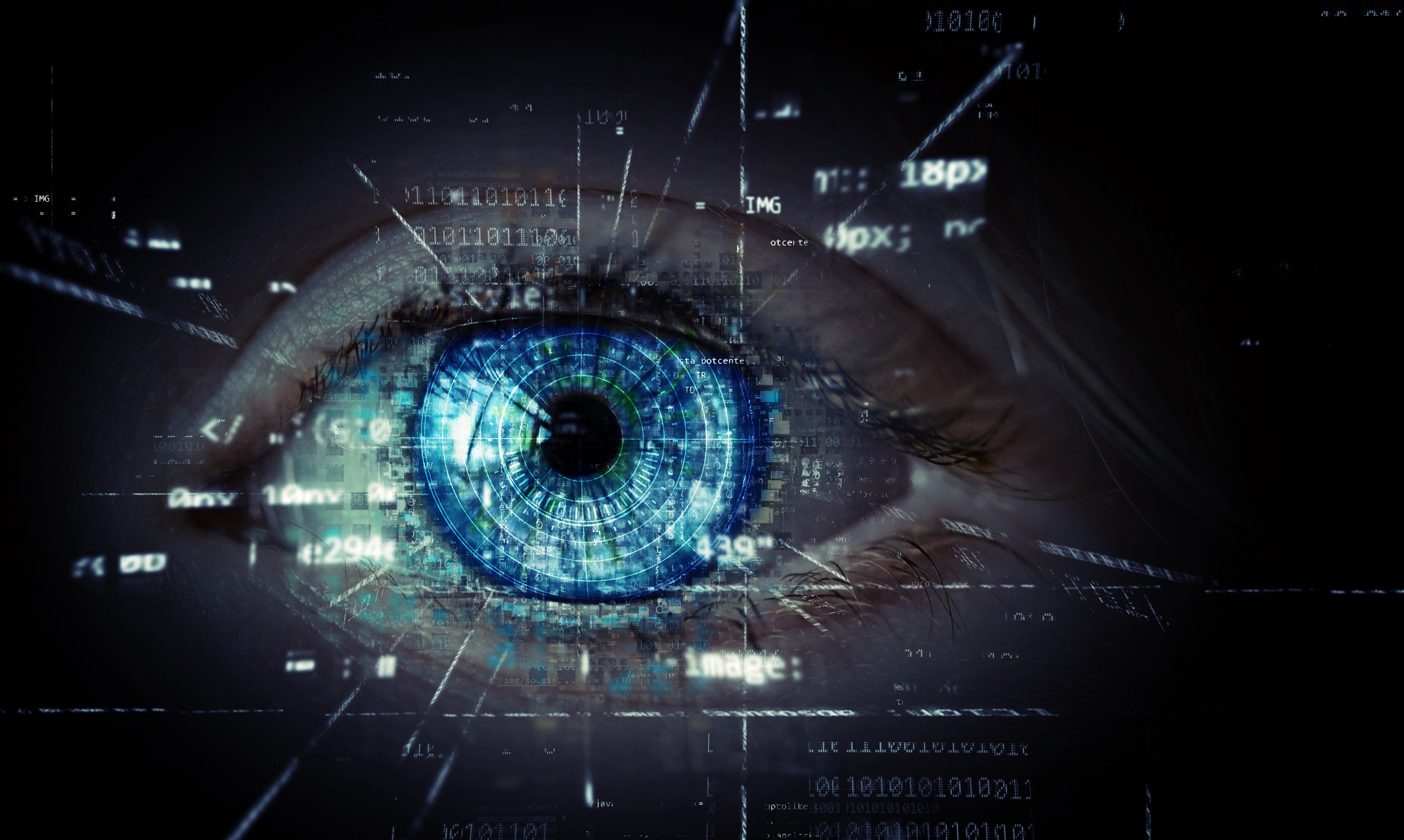
Domain Generalized Semantic Segmentation for LiDAR Point Clouds
– Published Date : 2023
– Category : Domain Generalization
– Place of publication : 제35회 영상 처리 및 이해에 관한 워크샵(IPIU)
Abstract:
With the success of 3D deep learning models, various LiDAR perception technologies for autonomous driving have been developed. While these models perform well in the source domain, they struggle in unseen target domains where domain gap exists. In this paper, we propose a domain generalization method for LiDAR semantic segmentation (DGLSS) that aims to ensure good performance for both source domain and unseen domains by only learning from a single source domain. We mainly target the domain shift from different LiDAR sensor configurations and scene distribution. To this end, we augment the source domain by randomly subsampling the LiDAR scans. We also introduce two constraints for generalizable representation learning: sparsity invariant feature consistency (SIFC) and semantic correlation consistency (SCC). SIFC aligns sparse internal features of the source domain with the augmented domain based on the feature affinity. For SCC, correlations between class prototypes within the source and the augmented domain are constrained to be similar. We establish a new domain generalization setting for training and evaluating. With the proposed evaluation setting, our method showed improved performance in the unseen domains compared to other baselines.