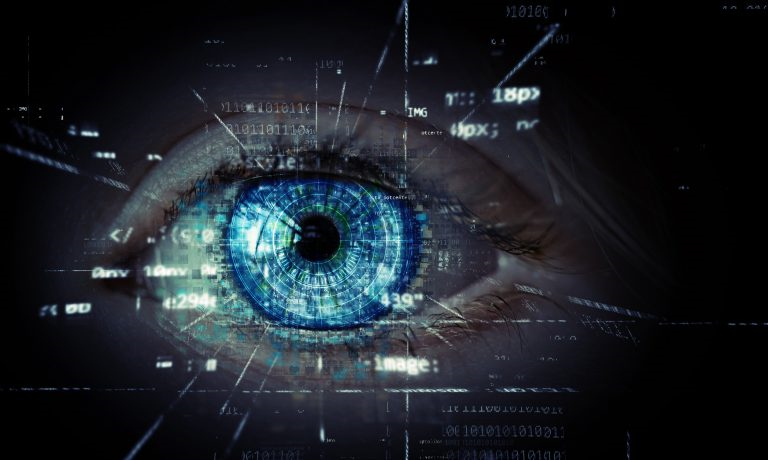
Polyp Detection via Imbalanced Learning and Discriminative Feature Learning
– Published Date: May 18, 2015
– Category: Tracking and Detection
– Place of publication: IEEE Transactions on Medical Imaging
Abstract
Recent achievement of the learning based classification leads to the noticeable performance improvement in automatic polyp detection. Here, building large good datasets is very crucial for learning a reliable detector. However, it is practically challenging due to the diversity of polyp types, expensive inspection, and labor- ntensive labeling tasks. For this reason, the polyp datasets usually tend to be imbalanced, i.e. the number of non polyp samples is much larger than that of polyp samples, and learning with those imbalanced datasets results in a detector biased toward a non polyp class. In this paper, we propose a data sampling based boosting frameworkto learn an unbiased polyp detector from the imbalanced datasets. In our learning scheme, we learn multiple weak classifiers with the datasets rebalanced by up/down sampling, and generate a polyp detector by combining them. In addition, for enhancing discriminability between polyps and non-polyps that have similar appearances, we propose an effective feature learning method using partial least square analysis, and use it for learning compact and discriminative features. Experimental results using challenging datasets show obvious performance improvement over other detectors. We further prove effectiveness and usefulness of the proposed methods with extensive evaluation.