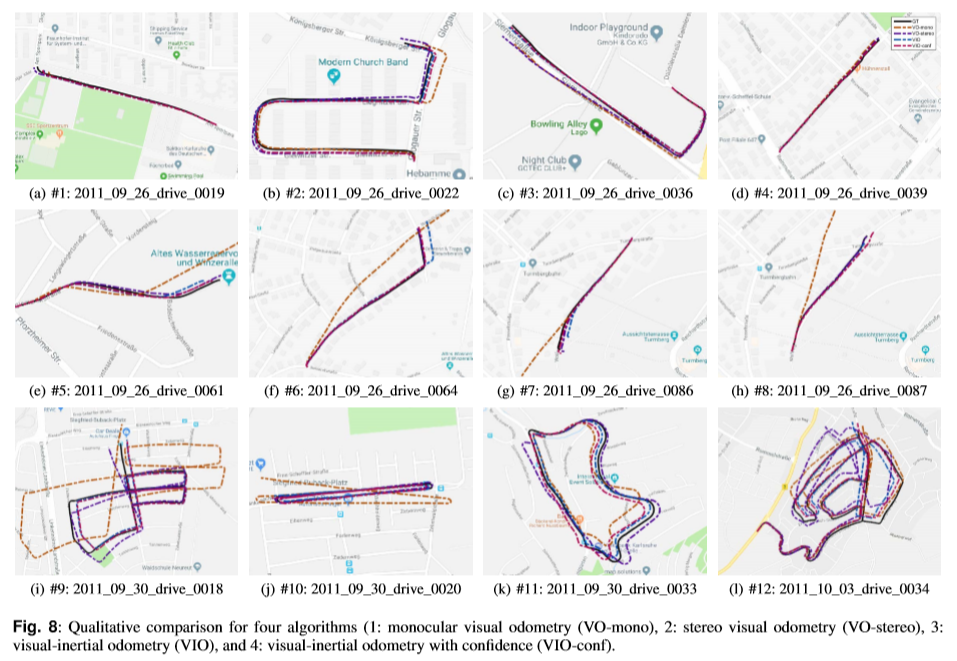
Confidence Analysis of Feature Points for Visual-Inertial Odometry of Urban Vehicles
– Published Date : July 2019
– Category : Visual-Inertial Odometry
– Place of publication : IET Intelligent Transport Systems
Abstract:
Visual-Inertial Odometry (VIO) estimate ego-motion using a camera and Inertial Measurement Unit (IMU). It shows outstanding performance for estimating ego-motion of a vehicle in absolute scale level thanks to the aid of the gyroscope and the accelerometer. However, in large-scale outdoor environments, the VIO has some difficulties in estimating translation because feature points along the motion direction and distant features points in the images cause degenerate situations. To solve these difficulties, we propose to infer the confidence of feature points and to elegantly incorporate the confidence to the Kalman filter based VIO. The confidence is computed from motion direction and displacements of tracked feature points under our urban canyon prior, and it is applied in cases that camera is moving forward to measurement noise covariances of the Kalman filter for ego-motion estimation. Experimental results on the public KITTI dataset show that the VIO outperforms monocular and stereo visual odometries, and the proposed VIO with confidence analysis achieves 1.82% translation error and 0.0018 deg/m rotation error.