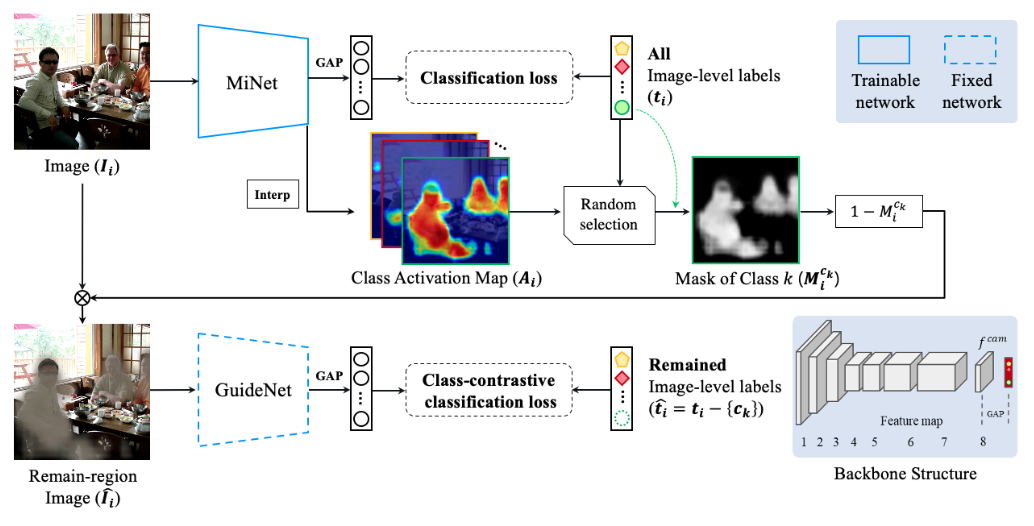
MiCAM: Mining Class Activation Maps with Image-level Supervision for Weakly Supervised Semantic Segmentation
– Published Date : 2021/02/03
– Category : Weakly-Supervised Semantic Segmentation
– Place of publication : The 33rd Workshop on Image Processing and Image Understanding (IPIU 2021)
Abstract:
The weakly-supervised semantic segmentation methods with image-level classification labels usually utilize the Class Activation Maps (CAMs) to localize objects of interest. While pointing out that CAMs are only activated on the most discriminative regions, adversarial erasing methods are proposed to further explore the less discriminative regions and overcome the under-activation problem. However, due to the lack of reliable guidance, such methods struggle with an over-erasing problem, a gradual expansion of activation regions to undesirable regions. We find that the classification network already has the capability to classify partially masked objects correctly, and therefore the classifier can be utilized as reliable guidance for adversarial erasing methods. In this paper, we propose a novel adversarial erasing framework that can effectively mine the object regions with the pre-trained classifier. In our framework, we design a network to generate a back-propagable mask of the randomly selected class in the label such that the pre-trained classifier cannot find that class from the masked image. By punishing the CAMs not to invade the object regions of other classes, our framework takes both advantages of preventing under-activation and over-erasing problems while generating CAMs with sharp boundaries. Along with the pseudo-labels refined from our CAMs and newly proposed re-train technique, we achieve the state-of-the-art on both PASCAL VOC 2012 val/test dataset only with image-level supervision.