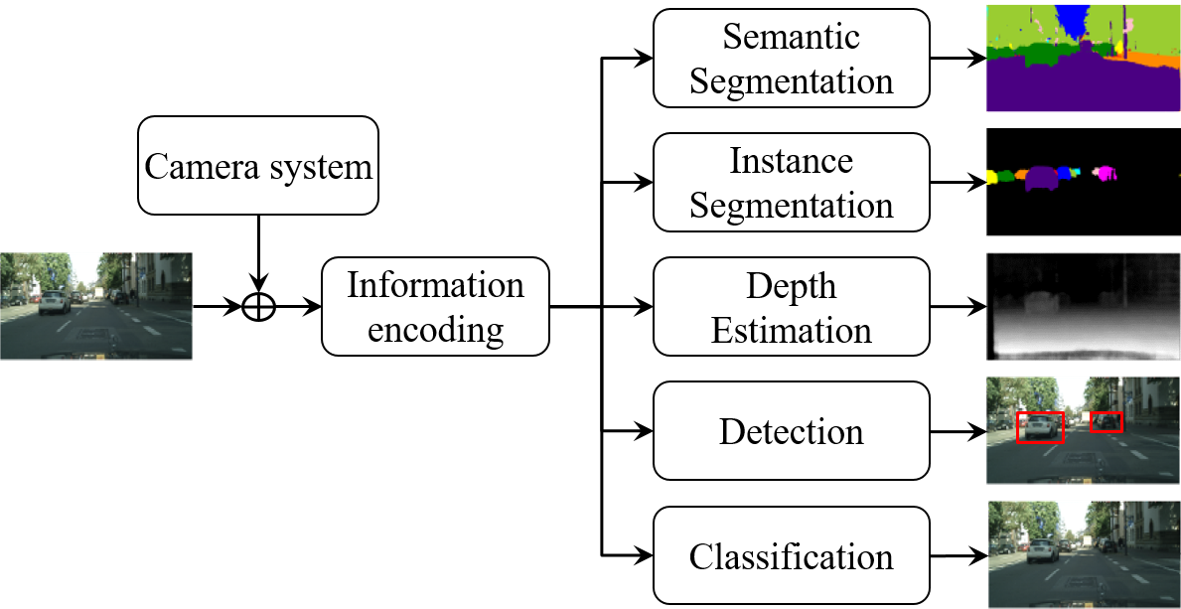
PSAT-GAN: Efficient Adversarial Attacks against Holistic Scene Understanding
– Published Date : TBD
– Category : Adversarial Attacks
– Place of publication : IEEE Transactions on Image Processing
Abstract:
Recent advances in deep neural networks (DNNs) have facilitated high-end applications, including holistic scene understanding (HSU), in which many tasks run in parallel with the same visual input. Following this trend, various methods have been proposed to use DNNs to perform multiple vision tasks. However, these methods are task-specific and less effective when considering multiple HSU tasks. End-to-end demonstrations of adversarial examples, which generate one-to-many heterogeneous adversarial examples in parallel from the same input, are scarce. Additionally, one-to-many mapping of adversarial examples for HSU usually requires joint representation learning and flexible constraints on magnitude, which can render the prevalent attack methods ineffective. In this paper, we propose PSAT-GAN, an end-to-end framework that follows the pipeline of HSU. It is based on a mixture of generative models and an adversarial classifier that employs partial weight sharing to learn a one-to-many mapping of adversarial examples in parallel, each of which is effective for its corresponding task in HSU attacks. PSAT-GAN is further enhanced by applying novel adversarial and soft-constraint losses to generate effective perturbations and avoid studying transferability. Experimental results indicate that our method is efficient in generating both universal and image-dependent adversarial examples to fool HSU tasks under either targeted or non-targeted settings.