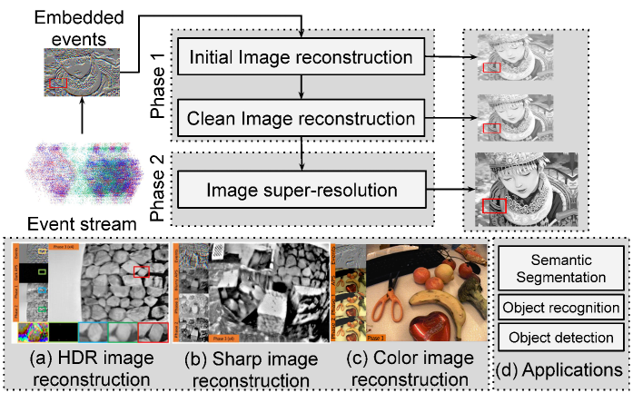
Joint Framework for Single Image Reconstruction and Super-Resolution with an Event Camera
– Published Date : TBD
– Category : Event Camera, Image Reconstruction, Super-Resolution
– Place of publication : IEEE Transactions on Pattern Analysis and Machine Intelligence (TPAMI)
Abstract:
Event cameras sense brightness changes at each pixel and yield asynchronous event streams instead of producing intensity images. They have distinct advantages over conventional cameras, such as high dynamic ranges (HDR) and no motion blur. To take advantage of event cameras with existing image-based algorithms, some methods have been proposed to reconstruct images from event streams. The output images are, however, in a low resolution (LR) and unrealistic. The low-quality outputs stem from broader applications of event cameras, where high-quality and high-resolution (HR) images are needed. In this work, we consider the problem of reconstructing and super-resolving images from LR events when no ground truth (GT) HR images and degradation models are available. We propose a novel end-to-end joint framework for single image reconstruction and super-resolution from LR event data. Our method is primarily unsupervised to cope with GT’s absence of real inputs, deploying adversarial learning. To train our framework, we construct an open dataset including the simulated events and real-world images. The use of the dataset boosts up the network performance, and the network architectures and various loss functions in each phase help improve the result image qualities. Our experiments for image reconstruction show that our method surpasses the state-of-the-art LR image reconstruction methods for real-world and synthetic datasets. The experiments for SR image reconstruction also substantiate the effectiveness of our method. We further extend our method to more challenging problems of HDR, sharp image reconstruction, and color events. As an additional contribution, we demonstrate that the reconstruction and super-resolution results serve as intermediate representations of events for high-level tasks, such as semantic segmentation, object recognition, and detection. We further examine how events affect the outputs of three phases and analyze our method’s efficacy in an ablation study.