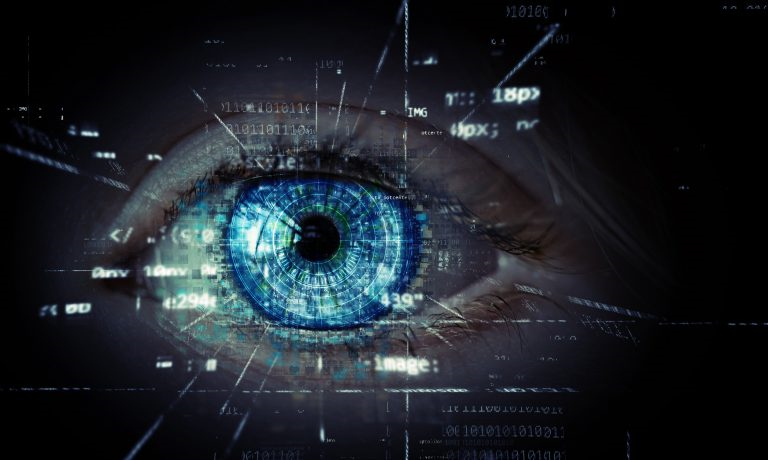
Ultra-Low-Power E-Noise System Based on Multi-Micro-LED-Integrated, Nanostructured Gas Sensors and Deep Learning
– Published Date : 2023
– Category : Deep Learning Application
– Place of publication : ACS NANO
Abstract:
As interests in air quality monitoring related to environmental pollution and industrial safety increase, demands for gas sensors are rapidly increasing. Among various gas sensor types, the semiconductor metal oxide (SMO)-type sensor has advantages of high sensitivity, low cost, mass production, and small size but suffers from poor selectivity. To solve this problem, electronic nose (e-nose) systems using a gas sensor array and pattern recognition are widely used. However, as the number of sensors in the e-nose system increases, total power consumption also increases. In this study, an ultra-low-power e-nose system was developed using ultraviolet (UV) micro-LED (μLED) gas sensors and a convolutional neural network (CNN). A monolithic photoactivated gas sensor was developed by depositing a nanocolumnar In2O3 film coated with plasmonic metal nanoparticles (NPs) directly on the μLED. The e-nose system consists of two different μLED sensors with silver and gold NP coating, and the total power consumption was measured as 0.38 mW, which is one-hundredth of the conventional heater-based e-nose system. Responses to various target gases measured by multi-μLED gas sensors were analyzed by pattern recognition and used as the training data for the CNN algorithm. As a result, a real-time, highly selective e-nose system with a gas classification accuracy of 99.32% and a gas concentration regression error (mean absolute) of 13.82% for five different gases (air, ethanol, NO2, acetone, methanol) was developed. The μLED-based e-nose system can be stably battery-driven for a long period and is expected to be widely used in environmental internet of things (IoT) applications.