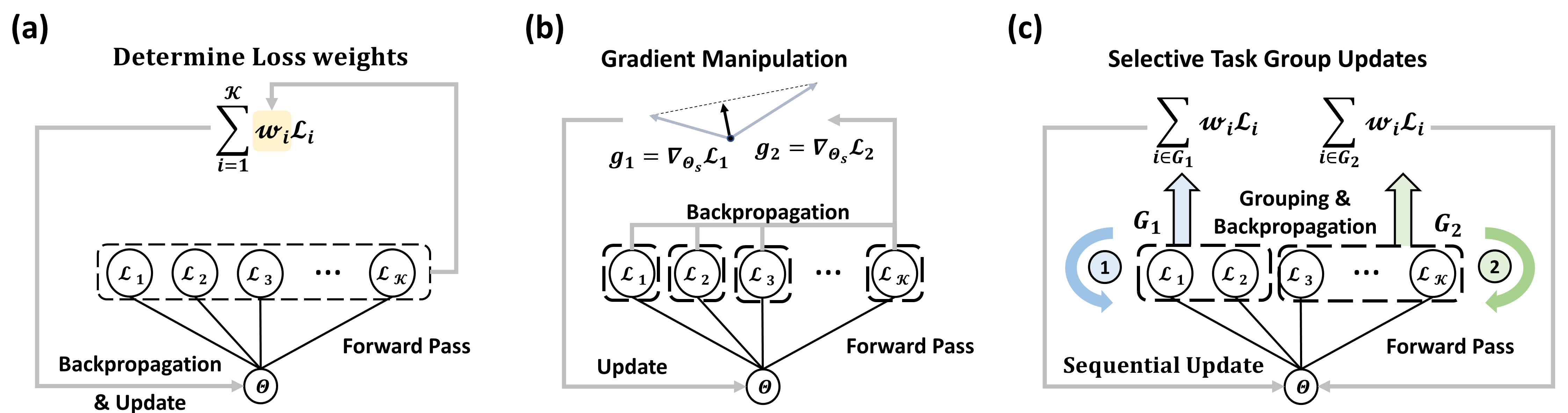
Selective Task Group Updates For Multi-Task Optimization
– Published Date : 2025.04.24
– Category : Multi-Task Learning, Optimization
– Place of publication : 13th International Conference on Learning Representations (ICLR 2025)
Abstract:
Multi-task learning enables the acquisition of task-generic knowledge by training multiple tasks within a unified architecture. However, training all tasks together in a single architecture can lead to performance degradation, known as negative transfer, which is a main concern in multi-task learning. Previous works have addressed this issue by optimizing the multi-task network through gradient manipulation or weighted loss adjustments. However, their optimization strategy focuses on addressing task imbalance in shared parameters, neglecting the learning of task-specific parameters. As a result, they show limitations in mitigating negative transfer, since the learning of shared space and task-specific information influences each other during optimization. To address this, we propose a different approach to enhance multi-task performance by selectively grouping tasks and updating them for each batch during optimization. We introduce an algorithm that adaptively determines how to effectively group tasks and update them during the learning process. To track inter-task relations and optimize multi-task networks simultaneously, we propose proximal inter-task affinity, which can be measured during the optimization process. We provide a theoretical analysis on how dividing tasks into multiple groups and updating them sequentially significantly affects multi-task performance by enhancing the learning of task-specific parameters. Our methods substantially outperform previous multi-task optimization approaches and are scalable to different architectures and various numbers of tasks.