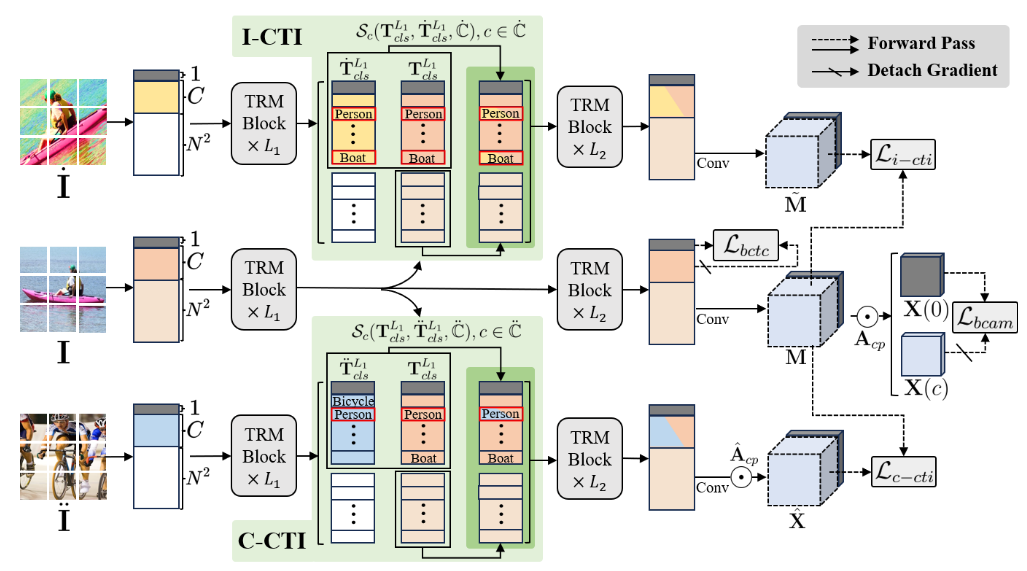
Class Tokens Infusion for Weakly Supervised Semantic Segmentation
– Published Date : TBD
– Category : Weakly Supervised Semantic Segmentation
– Place of publication : IEEE/CVF Conference on Computer Vision and Pattern Recognition (CVPR) 2024
Abstract:
Weakly Supervised Semantic Segmentation (WSSS) relies on Class Activation Maps (CAMs) to extract spatial information from image-level labels. With the success of Vision Transformer (ViT), the migration of ViT is actively conducted in WSSS. This work proposes a novel WSSS framework with Class Token Infusion (CTI). By infusing the class tokens from images, we guide class tokens to possess class-specific distinct characteristics and global-local consistency. For this, we devise two kinds of token infusion: 1) Intra-image Class Token Infusion (I-CTI) and 2) Cross-Image Class Token Infusion (C-CTI). In I-CTI, we infuse the class tokens from the \underline{same} but differently augmented images and thus make CAMs consistent among various deformations (\ie view, color). In C-CTI, by infusing the class tokens from the \underline{other} images and imposing the resulting CAMs to be similar, it learns class-specific distinct characteristics. Besides the CTI, we bring the background (BG) concept into ViT with the BG token to reduce the false positive activation of CAMs. We demonstrate the effectiveness of our method on PASCAL VOC 2012 and MS COCO 2014 datasets, achieving state-of-the-art results in weakly supervised semantic segmentation.